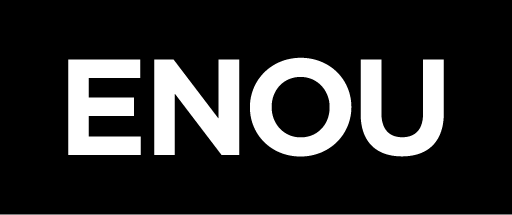
Technology solutions for the next generation of founders
Welcome to ENOU Labs LLC, the company behind Hapy Co, Hapy Design, and ShopSmith. We are dedicated to pushing the boundaries of digital development, design, and e-commerce, creating cutting-edge solutions that drive success for our clients.
Our Products
At ENOU Labs, we offer a range of specialized services through our distinct brands
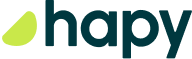
Hapy Co
Our digital development powerhouse, providing comprehensive product development solutions from concept to deployment. Visit Site
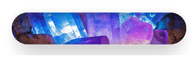
Hapy Design
Our creative design agency, specializing in user-centric web and mobile app designs, branding, and design systems. Visit Site
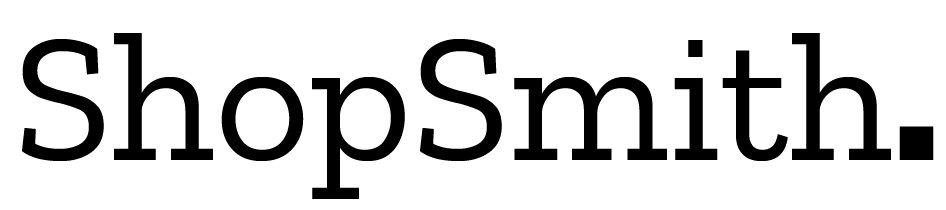
ShopSmith
Our e-commerce solution company, delivering robust, scalable online shopping platforms tailored to your business needs. Visit Site
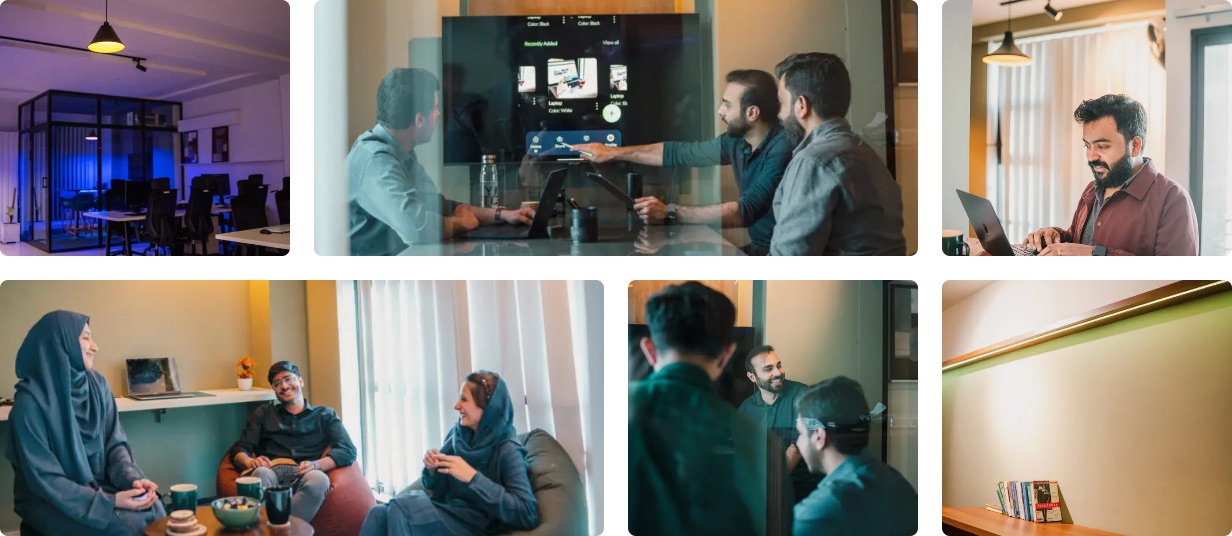
e. hello@enou.co
e. tahseen.khan@hapy.co
p. +14152555995
p. +923213434362
a. 2055 Limestone Rd, STE 200C Wilmington, Delaware, 19808, US